Learning from the Past (Digital Adoption) for the AI Future
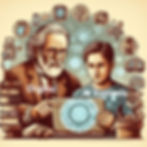
Over the last ten years, bringing digital stuff into companies showed instrumental lessons about technology, organisations, culture, and responsible innovation. Now, while AI promises big advances beyond digital, turning ideas into real solutions faces familiar obstacles that have tripped up efforts in the past.
Looking back at the digital history gives an AI roadmap for responsible adoption moving ahead.
Early on, digital dreams focused on mobility, connectivity and efficiency. But, there were many underestimated challenges in integrating and monitoring performance after launch in the real world. Similarly, AI’s fixation on accuracy and the content it creates can be distracting. The remaining work is shifting promising ideas into reliable solutions to business problems.
In the past, things like fragmented systems, scattered analytics and access gaps hindered digital success. But wins did happen by linking up platforms, IT and business data and setting some standards. Access to isolated data or black box modeling won’t cut it alone for AI. Jointly managing data, embedding predictions into real staff workflows, and aligning on AI rules are just as important as digital to internal partnerships across technology, culture and protocols.
There were large gaps between system abilities and employee comfort with digital, which were addressed through lots of training. Like those earlier tools, AI recommendations face adoption struggles without support. Explaining how the models work, offering data skills and transparency around changes—this is what builds confidence to really use AI instead of shying away from unfamiliar capabilities. Shortening the learning curve is crucial to match user readiness with AI potential when companies roll out these advanced technologies. Failing to prepare staff risks abandoned investments.
Yes, AI talent is scarce. But priorities need to stay on transparent, fair algorithm design guardrails—earning trust again like with digital security and privacy. Moreover, for AI to be comprehensive and unbiased, it depends on complete, quality data. Care in these areas also kept past digital efforts on track and remains vital for AI.
Digital success set benchmarks that aligned cultural and process priorities with technology resolving real issues—not just launching features. AI should similarly focus more on driving benefits over accuracy goals, whether business value or innovations that enhance decisions and lives.
Responsible AI adoption deserves focus now to sidestep digital growing pains. Digital did unlock the enterprise intelligence era, but there is still more potential left untapped. That’s the opportunity for AI advancements building on those lessons—turning ideas into robust solutions, teaming up around priorities, and measuring what matters most, ethically.
It is a matter of fact that AI innovation continues advancing rapidly. However, simply assuming algorithmic systems can smoothly move into business practices risks repeating adoption missteps around sustainability, governance, skills, and culture, as happened with digital adoption.
When it comes to AI, the most appropriate thing is keeping real people at the centre. That means clearly informing folks how these systems will impact their lives and jobs.
Get them skilled up through plenty of training, too. Make sure AI solutions actively include humans every step of the way—designing, monitoring, and collaborating. Protect people from harm by looking out for risks early. This is how companies can champion a truly human-focused approach to AI. It’s about empowering real humans, not replacing them.
Common sense puts people first. Leadership makes sure of it with AI.